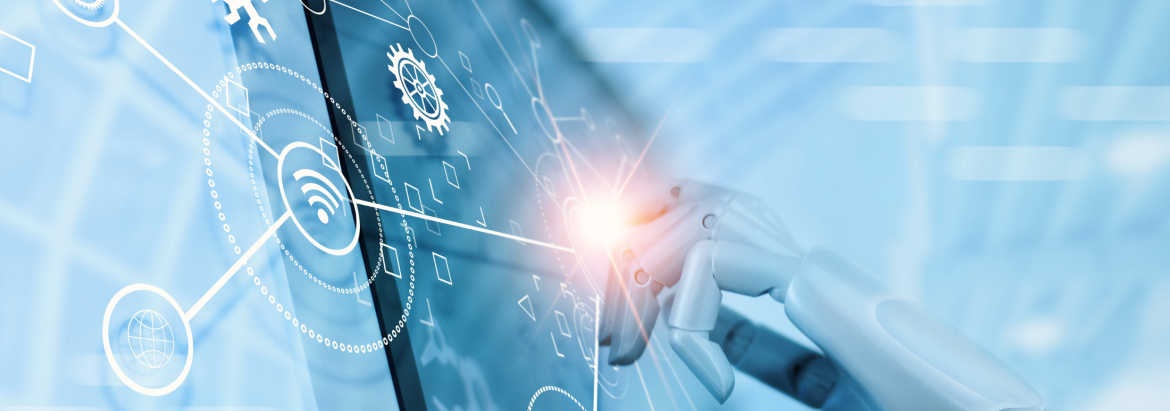
Understanding Causal AI and Its Impact on Enterprises
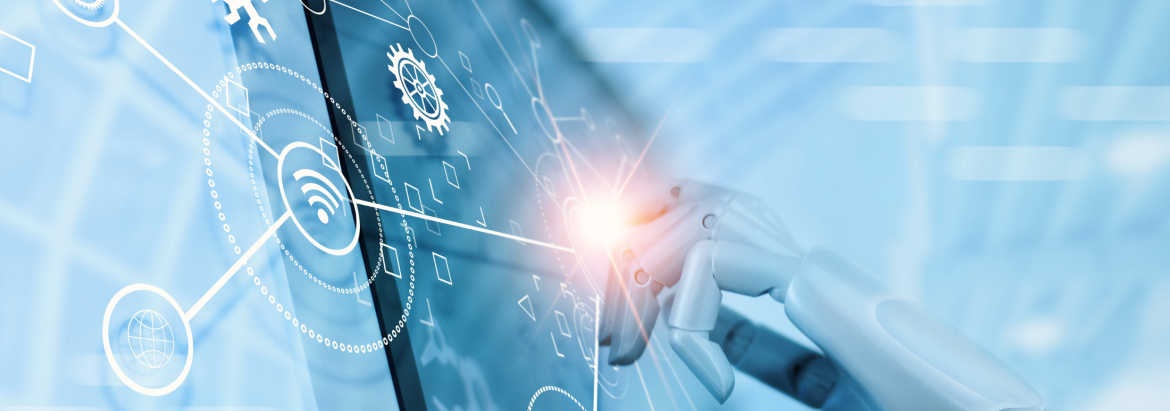
Enterprises keep an eye on the latest technologies that can increase the efficiency of operations, service availability, and customer satisfaction. In the past few years, enterprises are increasingly focusing on AI-based technology. Since enterprises are planning for hyper-automation, the role of AI is crucial. For example, more and more enterprises are investing in AIOps platforms for enhanced monitoring and security. Similarly, entities within the healthcare sector have started investing in generative AI for improved medical imagery. Among all the AI-based technologies, causal AI is gaining hype quickly. It has immense possibilities and can make choices similar to humans. Read on to understand more about causal AI and its impact on enterprises.
Sometimes, traditional rule-based technologies can make biased decisions. Due to the biased decision, the enterprise might lose its service availability. In such a situation, causal AI is the technology enterprises can count on. It has reasoning capabilities on par with humans and makes the right decisions. It can help organizations make rational decisions and understand their impact. Traditional rule-based models only identify how different events are related to each other. On the other hand, causal AI can determine the individual cause of each event. It will give a deeper meaning to how each event is related to others.
Causal AI understands that an event cannot occur on its own. It understands that something must have led to a particular event. It starts associating some variables to each event. The variables are the reason why a particular event has occurred. When performing a test, causal AI separates all the variables that aren’t related to an event. It leaves causal AI with the variables responsible for a particular event. While this might seem a very basic definition, it is exactly what causal AI does. Let us understand the concept of causal AI with a basic example.
Let us say a traditional rule-based model is given a dataset containing records of cold drink consumption, temperature, and wind speed. A traditional rule-based model might interpret that cold drink consumption increases with wind speed. As a human, we know that this is not true in the case of cold drink consumption. As a result, we have to help it understand that there is no relation between cold drink consumption and wind speed. On the other hand, causal AI can understand that cold drink consumption increases with the temperature rise. All this can be done without the need for human interaction.
Causal AI will determine a relationship between cause and effect. Where traditional rule-based models offer limited insights, causal AI can offer insights on a granular level. Not to forget, causal AI is less vulnerable to bias, compared to traditional rule-based models. Let us understand what impact causal AI has left on enterprises.
Causal AI helps enterprises in the journey from correlation to causation. When enterprises will know what has caused a particular event, they will have more chances to boost service availability. The cause-effect relationship produced by causal AI will be easy for humans to understand. Enterprises often struggle because their employees cannot understand how a certain outcome was produced. It creates doubt in their minds, and they might not trust their rule-based models. On the other hand, causal AI produces a human-like analysis of why it has reached a particular conclusion. The cause-effect relationship is easy for employees to understand.
Whenever a new automation technology comes to the scene, there are ethical concerns. For example, people could not trust the reliability of AIOps tools when they first came into the picture. As of now, enterprises completely understood the role of AIOps tools in monitoring and security. Similarly, enterprises are concerned regarding high-risk business decisions. It is because they think that traditional models can produce biased results. Causal AI can be used for high-risk decisions because it produces more accurate results.
Many enterprises use causal AI to execute simulated events. “What if” scenarios are simulated, and possible outcomes are determined. If the outcomes aren’t favorable, enterprises will know about them in advance. It again allows enterprises to make better business decisions that can help them in the long run. Causal AI is a technology that can be trusted by enterprises to perform high-risk actions. In the era of automation, precision, and accuracy also has their value. You cannot think of boosting service reliability with biased technology. Besides the mentioned benefits of causal AI, there are many other advantages for enterprises.
Even though causal AI has many benefits, there are certain limitations. Causal AI models are only as good as they are trained by humans. Some enterprises might not have data experts who know much about training models. As a result, they might provide causal AI models with insufficient models. In such a case, a causal AI model can also produce biased results. Another limitation of causal AI is that it is still in the research phase. There are fewer commercial applications and software systems based on causal AI. Big corporations like Google and Microsoft only invest heavily in causal AI research. Other entities are waiting for large corporations to reach a breakthrough and make their life easier. Having said this, causal AI has immense capabilities and must be explored more by enterprises.
Causal AI is an emerging technology that can help enterprises understand the cause and effect of each event. It will enable enterprises to make better decisions, as they will understand its effects. Although, Causal AI is still developing it will get better with time. Look out for AIOps platforms with causal AI capabilities!
Please complete the form details and a customer success representative will reach out to you shortly to schedule the demo. Thanks for your interest in ZIF!