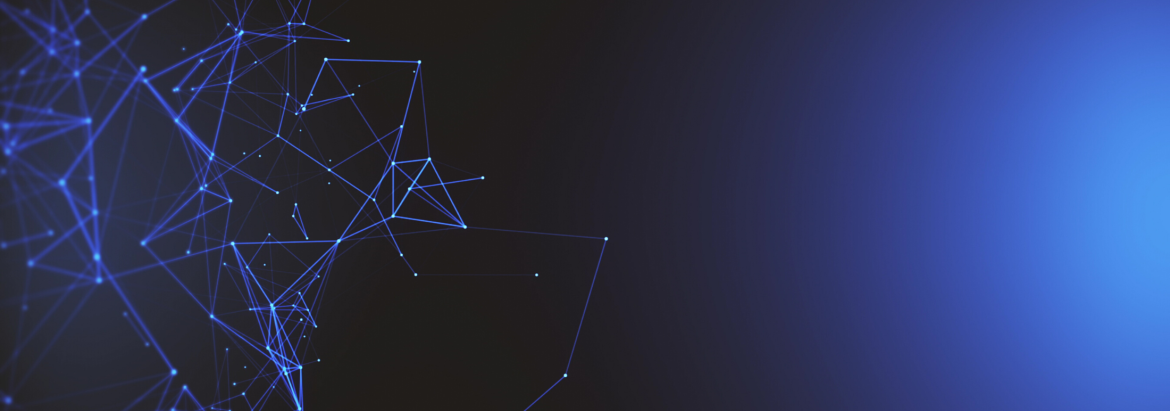
Understanding Big Data Management
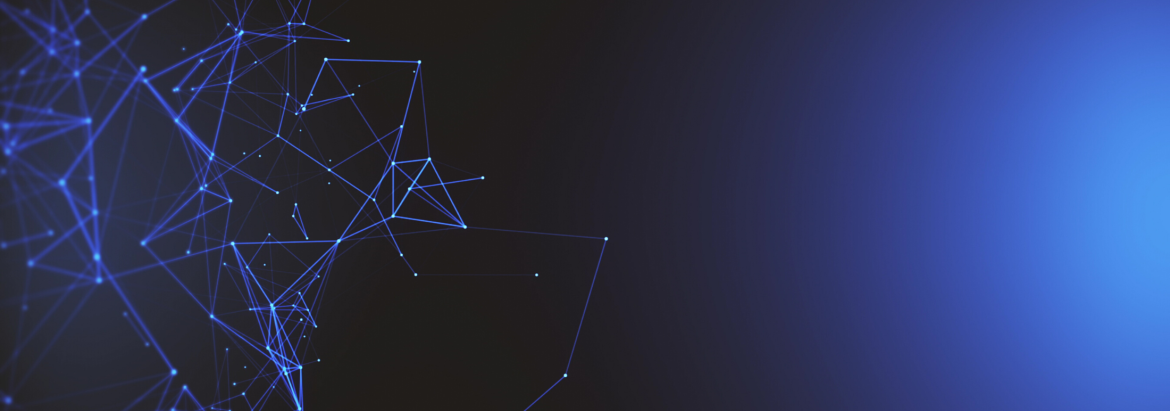
As the pace of digital transformation is increasing, more and more data is being produced. Enterprises produce large amounts of data daily around the world. Even though the data is unstructured when produced, enterprises cannot let it go into silos. There is a need to convert the data into a structured form and extract rich insights. For the same rationale, enterprises have started using efficient predictive analytics models. Since the volume of data is exponentially increasing, new techniques are used to reduce the manual labour for analysis. Considering the increasing volume of data, big data management is a must for enterprises. Read on to understand more about big data management, its architecture, and the best practices.
Big data management is handling and analyzing large amounts of structured and unstructured data. For enterprises, the data can be produced by software systems, business applications, customer devices, IoT devices, and many other sources. Traditional database management systems fail drastically in handling such large amounts of data. It is where big data management comes into the picture for storing, organizing, and processing large volumes of data. It is crucial to note that a large-sized organization will have large volumes of data to apply big data management strategies. Individuals will not invest in big data management for analyzing a few data sets.
Big data management prepares the data for business operations. Business intelligence and other processes count on big data management to access rich insights. At prima facie, one might not interpret anything from an unstructured data set. When the data set is organized and processed via big data management techniques, insights are produced. Insights are used by enterprises to make informed and better business decisions. A big data environment will allow analysts to work on relational databases, data warehouses, non-transactional data, and other fronts. Big data management environments also include technologies like predictive analytics models to perform high-end analysis.
Traditional database management systems also help with data analysis. One might debate the use of big data management in the presence of database management systems. Enterprises rely on big data management because it precedes traditional database management systems in many ways. Here’s why big data management is essential for organizations:
The architecture of big data management consists of data sources, big data platforms, and analytics tools. The first layer in big data management is the layer of data sources. It refers to different sources that generate large volumes of data. These sources could be anything, from customer smartphones to enterprise software systems.
The next layer in the architecture is the big data platform/environment. It consists of all the tools and technologies used to store, organize, and process large volumes of data. For example, it can include AIOps platforms used to analyze telemetry data. It can also include NoSQL databases, data processing frameworks, distributed file systems, and other tools or technologies. Together, these tools and technologies help in making the best use of available unstructured data.
The final layer of the big data architecture consists of analytical tools that can extract rich insights. It also includes data visualization tools, AI/ML frameworks, statistical solutions, and many other elements. The goal is to extract rich insights and represent them in an understandable form.
Here are some of the best big data management practices for enterprises in 2023:
New ways have emerged for handling large volumes of data, from AIOps platforms to causal AI. An enterprise must have a clear big data management strategy to extract valuable insights. These insights can be used for improved operations, cost-cutting, better business decisions, and more. Implement big data management techniques right away!
Please complete the form details and a customer success representative will reach out to you shortly to schedule the demo. Thanks for your interest in ZIF!