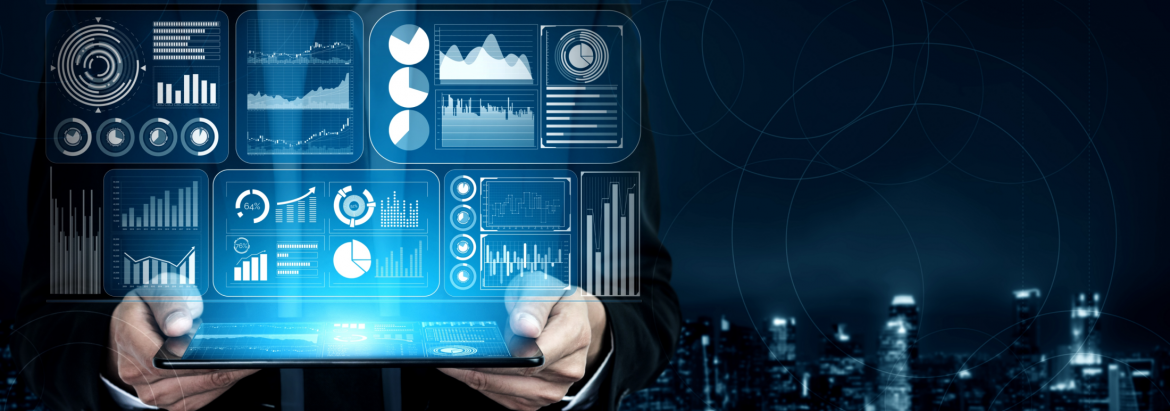
Predictive Analytics Techniques Which Prevent IT Outages
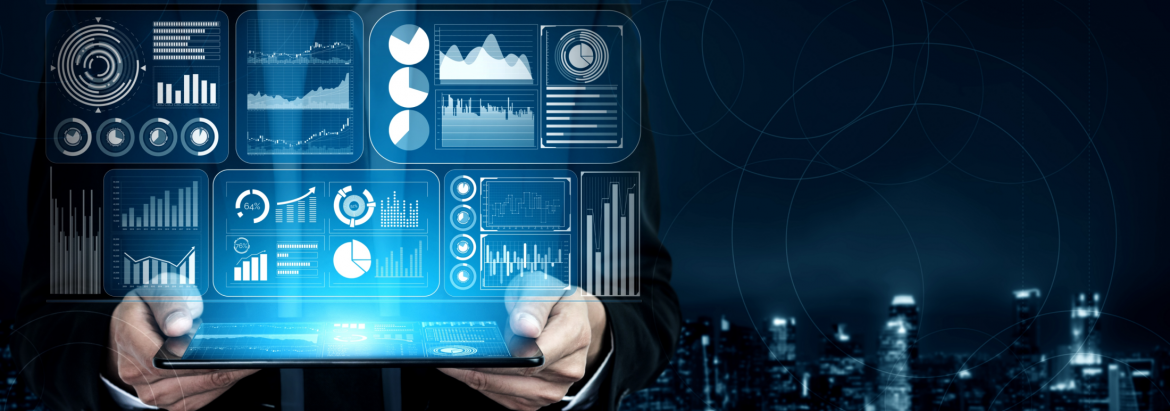
Unplanned IT downtime can cause a host of problems for IT teams to deal with: loss of valuable data, inability to maintain service continuity, vulnerability to security threats and an overall effect on business and revenue. Simultaneously, the availability of unprecedented data means that operational efficiency is directly dependent on the efficient management of this data. In recent times, predictive analysis is one of the best approaches to data mining. The insights generated via predictive analysis can help businesses make better decisions. ITOps (IT Operations) are adversely affected by system outages and they also affect the overall ROI (Return on Investment). Businesses have been leveraging the power of predictive analysis to gain useful insights to run their IT operations more efficiently
Some predictive analysis techniques that can prevent IT outages are:
For any dataset, the data range is decided with the help of dynamic thresholding. Static thresholding involves deciding data range manually which is not the case in dynamic thresholding. In dynamic thresholding, anomaly detection algorithms find the data range. The historical value of data points in a dataset is recorded by anomaly detection algorithms. The algorithms then train the data points with their historical value to find the data range. Once the normal data range is decided, anything outside it will be considered an anomaly.
Predictive analysis uses AI/ML algorithms that can predefine the range within which datapoints should exist. Abnormal values of data points can be easily known via predictive analysis. Since you can detect anomalies faster, you will reduce your MTTD (Mean Time to Detect). Reducing your MTTD will help you in preventing IT outages by acknowledging the incident faster.
In today’s software-driven world, organizations are producing large chunks of data every day. To detect an incident, the basic step is to find outliers within the data set. An outlier is a data point that shows abnormal values according to set norms. In multivariate anomaly detection, an outlier is decided as the combined score of at least two variables. For example, while mapping the sales and profit data points, an outlier can be decided with the combination of abnormal values of sales and profit. If a data point does not show a positive correlation between sales and profit then, that data point can be investigated.
Predictive analytics using AI applications will help an organization with multivariate anomaly detection. A predictive analytics platform will automatically fix the value of an outlier by combing two or more variables. Any data point then recognized as an outlier can be known and solved quickly. Faster anomaly detection will further help you in preventing IT outages.
Organizations must deal with sequential data all the time. Sequential data are data points in a dataset that is dependent on other data points in the same dataset. Sequential pattern analysis discovers subsequence in a dataset that can also be called a pattern in a dataset. Let us understand this by an example. Suppose that a customer tends to buy some products together from an e-commerce site. The products brought by the customer are recorded as follows:
Customer = ({1,2}, {2,3}, {4}, {5,6}, {6})
If 1,2,3,4,5,6 are products then you can see the sequence in the buying pattern of the customer. One can notice that the customer brought the product 1 and 2 together and product 4 alone. Similarly, when we talk about anomaly detection, sequential pattern analysis will determine the patterns within the datasets. An anomaly can be identified faster by sequential pattern analysis. If a relationship exists between two anomalies, it can also be known via sequential pattern analysis.
An organization may have many software systems that are responsible for essential business processes. Application performance monitoring is needed to make sure all the software systems are running as expected. However, traditional monitoring tools cannot predict capacity exhaustion. Capacity exhaustion is predicting when the software systems will expire or lose their ability to perform essential business processes. If you can predict capacity exhaustion, then you can take proactive steps to stop it.
Predictive analytics utilizes the historical usage of the IT infrastructure to predict capacity exhaustion. Previous system outages are also used by predictive algorithms to know the time of another system failure. If more load is experienced by software systems, predictive analysis can help CIOs/CTOs become aware and proactive. Organizations can purchase more capacity in advance to prevent any system failures. With modern predictive analytics models, one can add more bandwidth to software systems to stop IT outages.
Predictive analytics using AI applications will also help in uncovering potential risks and loopholes within the IT infrastructure. Cyber-attacks can shut down IT systems for a longer time. Predictive analytics tools detect any fraudulent activity within the organization. It also remembers each incident and pattern occurring during cyber-attacks. If the same cyberattack occurs twice, predictive analysis algorithms will identify it immediately.
Predictive analytics models perform rigorous monitoring of software systems. Any vulnerabilities within the IT infrastructure will be identified in advance via predictive analysis. By eliminating cyber threats, you can significantly decrease the chances of IT outages.
AIOps (Artificial Intelligence for IT Operations) can help you with automated predictive analysis. AIOps platforms automatically parse the data collected from multiple sources and make it ready for predictive analysis. AIOps platforms identify trends and patterns in the data set to identify outliers/anomalies. It will also provide you with actionable insights on how to solve an incident. Since you can detect and solve anomalies faster, you can decrease the chances of IT outages.
With predictive analytics, you can ensure better performance by forecasting resource utilization. It can help you predict ticket volumes, spikes, and resource utilization levels in advance. You can also predict incident volume and usage patterns to help you become proactive and avoid IT downtime. Further, predictive analytics can help organizations forecast potential failures and liabilities by identifying patterns and situations that precede an outage and then take preventive actions accordingly
Recent studies show that 45% of businesses are already using AIOps for better predictive analysis. An AIOps based analytics platform will also help you in slashing costs as you don’t have to hire more system administrators for rigorous monitoring. Choose AI for application monitoring and predictive analysis!
Please complete the form details and a customer success representative will reach out to you shortly to schedule the demo. Thanks for your interest in ZIF!