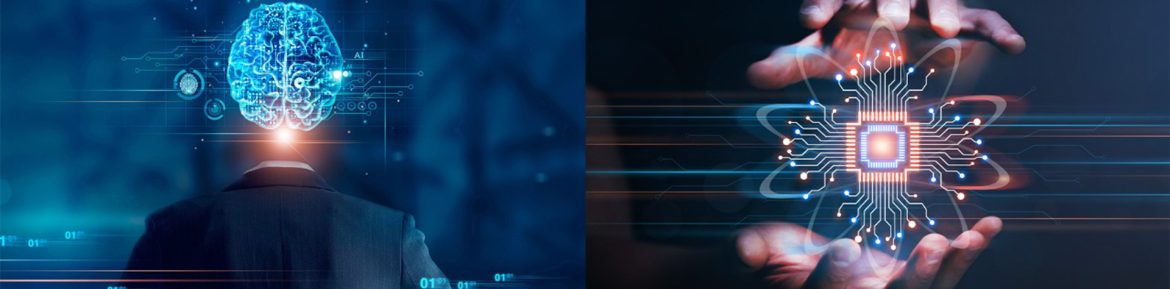
Is AI Gender-Biased?
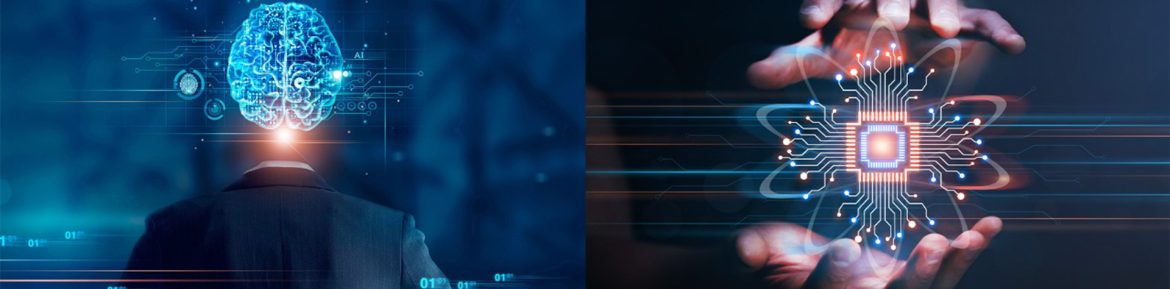
Did you know that algorithms could be sexist?! Unfortunately, it is true! As we celebrate International Women’s Day this month, there can’t be a better time to discuss such a topic.
According to Harvard Business Review, there have been many incidences of AI adopting gender bias from humans. It cites an example of natural language processing (NLP) that can be found in Amazon’s Alexa and Apple’s Siri. It not only affects women, but also business and economies to a large extent.
There is an agreement that adequate good data can indeed help close gender gaps, but the question is are the “right” questions being asked to “right” people in the data collection process.
During machine learning. It depends on the dataset being fed. If there’s not enough women contributing, then a woman’s perspective remains missing in the AI’s knowledge, and that leads to a biased algorithm. Machine learning is led by humans, which means their own bias will be incorporated within the AI system.
Those who make decisions informing AI systems and those who are on the team developing AI systems shapes their learning. And unsurprisingly, there is a huge gender gap: Only 22% of professionals in AI and data science fields are women—and they are more likely to occupy jobs associated with less status.
We are not discussing a mere theory of discrimination. AI bias could put a woman’s life in jeopardy based on the products and services they use. Let us analyze how.
Take the concrete example of seatbelts, headrests and airbags in cars which have been designed mainly based on data collected from car crash dummy tests using the physique of men and their seating position. Women’s breasts and pregnant bodies don’t feed into the ‘standard’ measurements. As a result, women are 47% more likely to be seriously injured and 17% more likely to die than a man in a similar accident explains Caroline Criado Perez, author of Invisible Women, and Lauren Klein, co-author of Data Feminism, in a recent BBC interview.
A gender gap in data is not always life threatening, but the design and use of AI models in different industries can significantly disadvantage women’s social status. Another incident of 2019 can be cited where a couple applied for the same credit card and the wife was set a credit limit of almost half the amount by the company despite having a creditworthiness almost equal to her husband.
Gender bias have been found in almost all industries – who the health care system prioritizes for COVID-19 vaccines, which candidate a company calls in for job interviews, how much credit financial institutions offer different customers, etc.
Medical studies which can exclude representative samples of women (including pregnant women, women in menopause, or women using birth control pills) may result in medical advice that is not necessarily suitable for the female body suggests Alexandra Kautzky-Willer, head of the Gender Medicine Unit at the Medical University of Vienna, Austria.
In addition to gender bias, AI faces another challenge – of racial bias. It raises concern when AI technology is supposed to diagnose skin cancer for which the accurate detection of skin color and its variances matter. Algorithmic Justice League founder Joy Buolamwini found that various face recognition algorithms considered 80% images of white persons and 75% male faces. As a result, the algorithms had a high accuracy of 99% in detecting male faces, but only 65% when it came to recognizing black women.
The first step towards overcoming bias is making sure training samples are as diverse as possible and the people developing AI are also from different backgrounds. The AI industry needs to work towards equality, both in its approach and perspective. AI companies need to employ more women in tech jobs, to diversify workforce creating these new technologies.
Let’s take cognizance of some stats on women in tech –
To better serve business and society, fighting algorithmic bias needs to be a priority. “By 2022, 85% of AI projects will deliver erroneous outcomes due to bias in data, algorithms or the teams responsible for managing them. This is not just a problem for gender inequality – it also undermines the usefulness of AI”, according to Gartner, Inc.
Personal biases should not get into the way of design. A gender-neutral approach in developing or marketing AI tech products, putting an end to psychological stereotyping such as female voices, female stereotypes, change in mindsets and attitudes, and also practices we can embed into our daily life could be a big leap towards what we are trying to achieve.
Bias may be an unavoidable factor of life, but steps can be taken to not introduce that into new technologies. New technologies give us a chance to start afresh – but it’s up to people, not the machines, to remove bias. So hopefully women, together with men, will play a large and critical role in shaping the future of a bias-free AI world.
These actions are not exhaustive, but they provide a starting point for building gender-smart ML that advances equity. Let’s not miss this opportunity to revolutionize how we think about, design, and manage AI systems and thereby offer a more just world for today and for future generations.
Please complete the form details and a customer success representative will reach out to you shortly to schedule the demo. Thanks for your interest in ZIF!