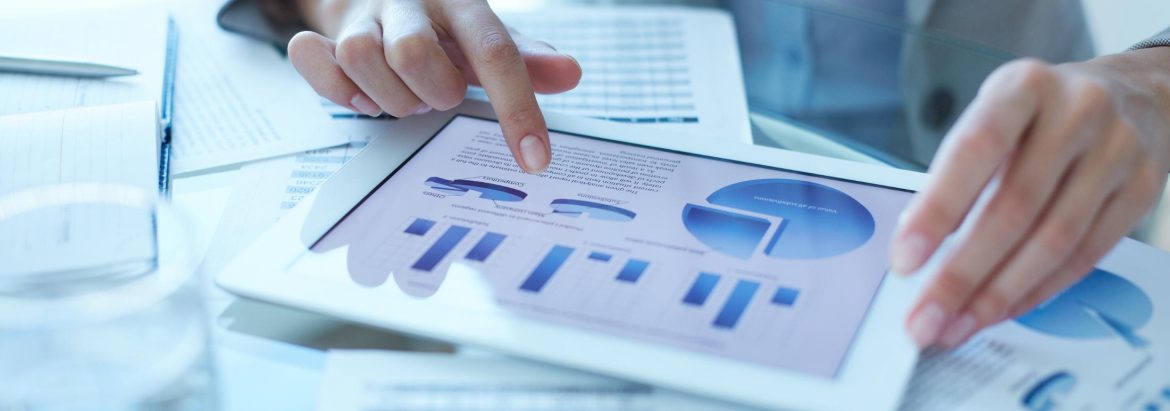
How to Improve the Accuracy of Predictive AIOps
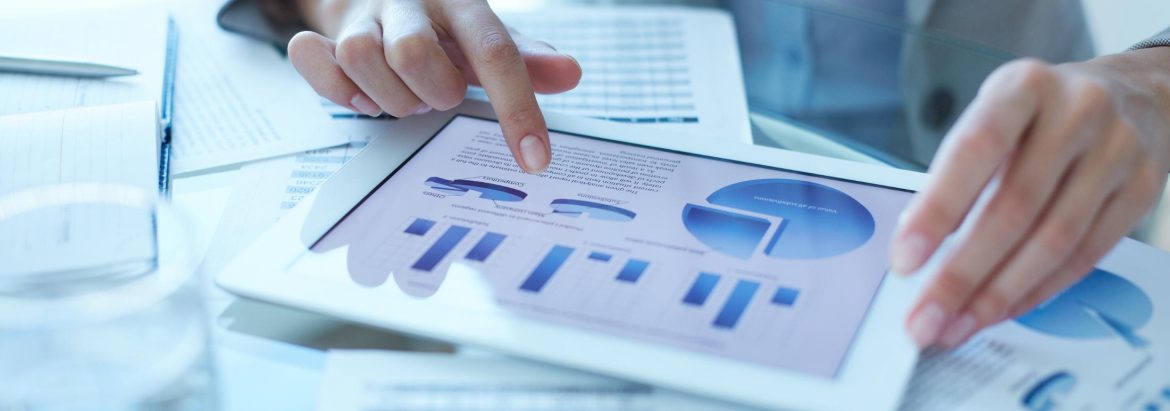
Every business organization believes in digital transformation. However, numerous challenges arise with digital transformation. The biggest challenge is to monitor the dynamic IT infrastructure responsible for providing IT services. If any incident within the IT infrastructure goes unnoticed, service availability could fall apart.
Most of the woes of CIO/CTOs were solved after the introduction of AIOps. AIOps-based monitoring solutions were capable of monitoring the dynamic IT infrastructure without any manual support. AIOps-based monitoring tools use predictive analytics models to identify service issues before they trigger performance degradation. Proactive monitoring of the IT infrastructure to prevent performance degradation is one of the biggest advantages of AIOps. However, business organizations should know ways to improve the accuracy of AIOps based analytics platforms. Read on to know ways to improve the accuracy of predictive AIOps.
Predictive analytics models based on AI/ML algorithms learn with time. You cannot expect an AIOps based analytics platform to start providing you with high-end insights right away. It takes some time for AI/ML algorithms to understand the environment, collect ample data, and react better next time. With each passing day, your AIOps-based monitoring solution will become better. However, there are certain steps that business organizations can take to boost the productivity of predictive AIOps. Once you have fully trained predictive AIOps, it will boost your overall service availability remarkably. Practices that can improve the accuracy of predictive AIOps are as follows:
Add training data:
IT teams should focus on training the AIOps based analytics platform with training data. Without substantial training data, predictive AIOps will never learn about their surroundings. Before installing predictive AIOps in your organization, provide it with historical data for improved predictions. Without ample data, an AIOps based analytics platform will only make assumptions. For monitoring teams, it will be like shooting in the dark.
For detecting performance issues, AIOps correlates system events. While event correlation, AIOps can use historical data from past years with ease. If AIOps is not provided with historical data, it will not have ample event data for correlation. Event correlation is important because it helps in identifying patterns that lead to performance degradation. Take some time to train your AIOps based analytics platform with training data to get the most out of it.
Add missing values:
While training your AIOps-based monitoring platform, make sure the dataset does not have any missing values. If your data set has missing values, predictive analytics models will not perform to their fullest. The best way to treat missing values is to replace them with the mean of all the data entities present in the data set. Many IT individuals treat the missing values by removing them from the data set. Some data analysts also remove outliers from the data set before feeding it to an AIOps-based monitoring solution. Well, removing any data entity from the data set is not a recommended action for IT monitoring teams.
Normalize the data:
Data normalizing is done to reduce redundant data and improve the integrity of the dataset. A large table representing any dataset can be distributed into several small tables via data normalization. IT teams believe in data normalization to make data analytics easier for predictive analytics models. With data normalization, the information will make more sense than before. AI/ML algorithms tend to work efficiently when there is a normal distribution of data. Make sure to normalize the entire data into the same scale before feeding it to AIOps based analytics platform.
Indulge in feature selection:
You need to select effective data attributes that can best explain the relationship between data entities. Relationships between the independent data entity are target data entity can be found easily when feature selection is done effectively. Choose a subset of attributes that can best explain the relationship between data entities. With effective feature selection, you can get the most out of predictive analytics models.
With the aforementioned practices, you can get the most out of your AIOps based analytics platform. When you train an AIOps-based monitoring solution, it will have the fuel it needs going forward. With the historical and current event data, predictive AIOps will identify performance issues. If the capacity of any software system is going to exhaust, predictive AIOps will inform you beforehand. When trained and provided with normalized data, predictive AIOps can help you increase service availability significantly.
How to know whether predictive AIOps is working perfectly?
How will you know whether predictive AIOps is performing to its full potential? Well, you can know about the effectiveness of predictive AIOps via several metrics. You can measure those metrics before you train your AIOps-based monitoring solution. The same metrics can then be measured after successfully training predictive analytics models. Some of the metrics that help you measure the effectiveness of predictive AIOps are as follows:
Gone are the days when only a few system administrators were enough for rigorous monitoring of the IT environment. At present, you need the power of AI along with expert employees to avoid performance degradation. Boost service availability with the power of predictive AIOps!
Please complete the form details and a customer success representative will reach out to you shortly to schedule the demo. Thanks for your interest in ZIF!