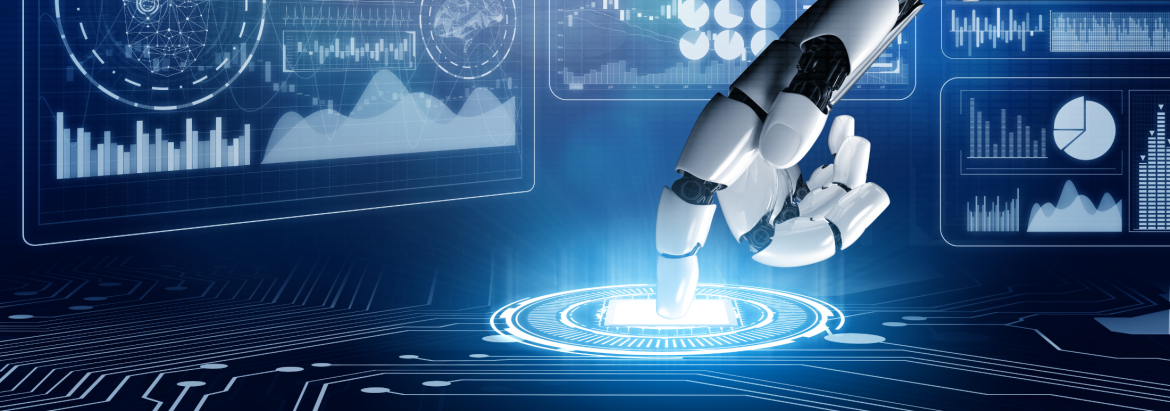
Causal Inference for Counterfactual Predictions in AI
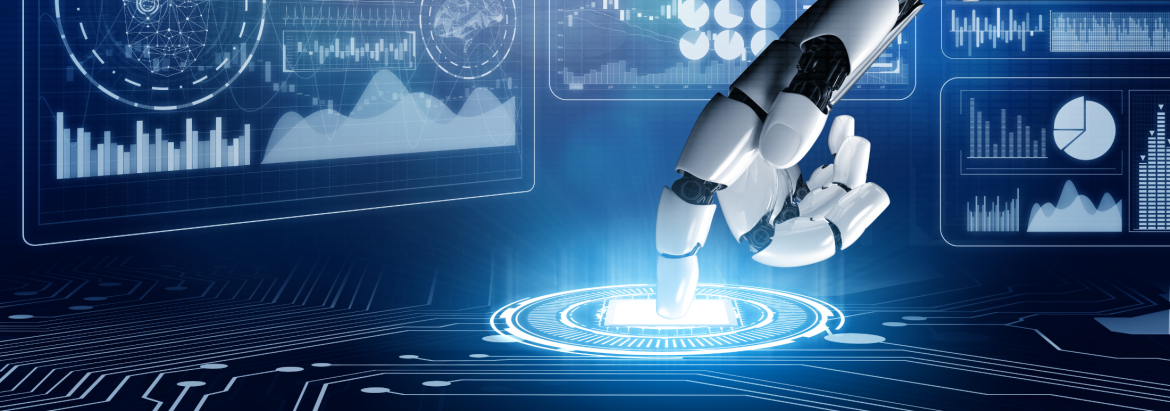
Advancements in AI have increased significantly over the years. In 2023, AI (Artificial Intelligence) is everywhere around us, from self-driving cars to chatbots. Corporate enterprises have also started using AI for operations, customer support, research, and many other processes. One of the main benefits of AI is that it can make predictions for events that haven’t occurred yet. Predictions via AI-led solutions allow enterprises to make informed decisions. Many enterprises are using AI-led solutions for eliminating IT incidents and improving service availability. Among different AI-led technologies, causal AI is making waves due to its capabilities. Enterprises are increasingly investing in simulations and counterfactual predictions in 2023. When it comes to accurate counterfactual predictions, causal AI is the way to go. Let us understand causal inference for counterfactual predictions in AI.
There can be situations producing multiple outcomes, which are not known to the AI system. Also, multiple variables could be involved in producing the outcomes. Traditional rule-based models cannot predict the right outcomes in such situations. They fail to produce the right result when there are multiple outcomes or variables. In such a case, causal inference comes into the picture to determine the right results. With the help of causal inference, relationships between different variables are determined. A causal AI system will understand how different variables interact with each other. The relationship produced via causal AI is not similar to that produced by traditional rule-based models. It is more of a causal relationship where you know what factors have led to a particular outcome.
Causal AI enhances the level of observability for enterprises. It is widely used in the industry to make accurate counterfactual predictions, which cannot be done by other branches of AI. Without understanding the causal relationships between different variables, one cannot make counterfactual predictions. Are you familiar with the concept of counterfactual predictions?
Counterfactual predictions are outcomes that would happen when certain variables are changed. It determines what will happen if certain conditions are changed. It is crucial to note that the original conditions of an event are not changed physically. The variables and conditions are hypothetically changed by a causal AI system, and outcomes are determined. A hypothetical scenario is created where the original conditions are changed, and causal AI is used to make predictions. It helps in situations where you cannot perform manual or practical tests after changing the original conditions of an event. For example, you cannot change the existing conditions repeatedly during medical trials. For the same rationale, healthcare entities use causal AI to make counterfactual predictions regarding medical trials.
For making counterfactual predictions, data analysts use a variety of causal techniques. These techniques help in understanding the causal relationships between variables. Experts use observational studies, randomised controlled trials, and natural experiments for identifying causal relationships between variables. Many new techniques are evolving, but these are the most effective ones for determining causal relationships. With the help of observational studies, outcomes of different events are recorded without changing the original variables. Once the outcomes are recorded, statistical methods are used to determine cause-effect relationships. Randomised controlled trials are actively used in the healthcare sector. It involves assigning candidates to different treatments and recording the outcomes. After outcomes are recorded, the most effective treatment is sorted out. Natural experiments are also used to determine causal relationships between different factors/variables. It involves recording the outcomes of events that occur naturally, without any manual interference.
Once the causal relationships between variables are established, it is time to make counterfactual predictions. Since the scenario is changed, predictions are made according to the new variables. For example, we all know that consuming tobacco might lead to mouth cancer. In such a case, causal inference can be used to predict what would happen if a person never consumed tobacco. One can also check what would happen if a person stops consuming tobacco after the first few years. Healthcare entities use causal inference to make counterfactual predictions for patients. For example, they can predict what would have happened if the patient received a different treatment.
Traditional rule-based models sometimes fail to provide accurate results. In such a case, causal inference can be used to improve the accuracy of predictive analytics models. With the help of causal inference, analysts can remove bias from counterfactual predictions. For example, let us say a patient is likely to possess a certain disease. With the help of causal inference, we can predict what would happen if the patient receives a different treatment. The most effective outcome can be applied to the patient for recovery. It can help healthcare entities develop customised treatment plans for patients. Also, the level of accuracy is high with causal inference. Even though the situations are hypothetical, predictive analytics models provide accurate results. In sectors such as healthcare, decisions are critical and causal inference provides the required level of accuracy.
Causal inference for counterfactual predictions is not only limited to the healthcare sector. The corporate sector also uses counterfactual predictions to determine the outcomes of its initiatives. The causal inference technique has already surpassed the accuracy of traditional rule-based models. Since causal AI technology is also evolving, the level of accuracy will only go uphill. Enterprises often have to provide manual inference to traditional rule-based models. The hyper-automation level cannot be achieved when analysts offer human intuition to rule-based models. With causal inference, the prediction process can be fully automated. As the use of AI systems is continuously increasing, corporate enterprises are bound to use causal AI for improved accuracy.
Enterprises might not have the funds to conduct manual tests to determine possible outcomes. It is why the importance of counterfactual predictions increases in healthcare, corporate, and other sectors. Where traditional rule-based models fail to offer accuracy, AI-led solutions like causal AI models do the job. However, you will require good-quality data to make accurate counterfactual predictions. Data analysts must also train causal AI models in the right way to improve accuracy. Invest in causal inference for accurate counterfactual predictions now!
Please complete the form details and a customer success representative will reach out to you shortly to schedule the demo. Thanks for your interest in ZIF!